School of Educational Foundations, Leadership and Policy Faculty Publications
Document Type
Article
Abstract
The present Monte Carlo simulation study adds to the literature by analyzing parameter bias, rates of Type I and Type II error, and variance inflation factor (VIF) values produced under various multicollinearity conditions by multiple regressions with two, four, and six predictors. Findings indicate multicollinearity is unrelated to Type I error, but increases Type II error. Investigation of bias suggests that multicollinearity increases the variability in parameter bias, while leading to overall underestimation of parameters. Collinearity also increases VIF. In the case of all diagnostics however, increasing the number of predictors interacts with multicollinearity to compound observed problems.
Copyright Statement
Post-print
Publisher's Statement
This is an Accepted Manuscript of an article published by Taylor & Francis in Communications in Statistics - Simulation and Computation on 07 Nov 2017, available online: http://www.tandfonline.com/10.1080/03610918.2017.1371750.
Repository Citation
Lavery, Matthew Ryan; Acharya, Parul; Sivo, Stephen A.; and Xu, Lihua, "Number of Predictors and Multicollinearity: What are their Effects on Error and Bias in Regression?" (2017). School of Educational Foundations, Leadership and Policy Faculty Publications. 14.
https://scholarworks.bgsu.edu/seflp_pubs/14
Publication Date
11-7-2017
Publication Title
Communications in Statistics - Simulation and Computation
Publisher
Taylor & Francis
DOI
https://doi.org/10.1080/03610918.2017.1371750
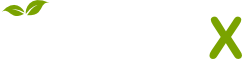
- Citations
- Citation Indexes: 131
- Usage
- Downloads: 3793
- Abstract Views: 33
- Captures
- Readers: 176
- Mentions
- Blog Mentions: 1